Capitule 5. Perfomance metrics
To measure the performance of a classifier is essential to know the phenomenon of the
field that you want to merge with ML to choose the correct performance metric. For example,
in the Brain-Computer interface field and according to Dr. Lotte, a metric that he suggests
is the area under the ROC curve.
A classical performance metric is the accuracy metric. Its function consists in counting the
correct patterns classified, remembering that we have two main subsets, i.e., training and testing.
All the performance metrics employ the values of the confusion matrix. The next figure is the
confusion matrix for two classes.

The next equation denotes the accuracy metric.
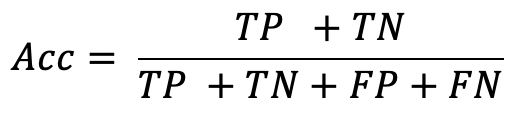
TPR (True Positive Rate ) and FPR (False Positive Rate) are denoted in the next equations.
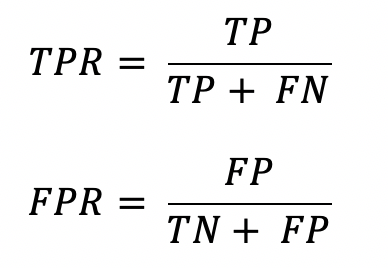
- Investigate which other performance metrics exist with its corresponding equation.
- Investigate the meaning of "False Positive" and "False Negative."
- Answer the next question and explain your answer: Is it correct to compare the metrics performance results?